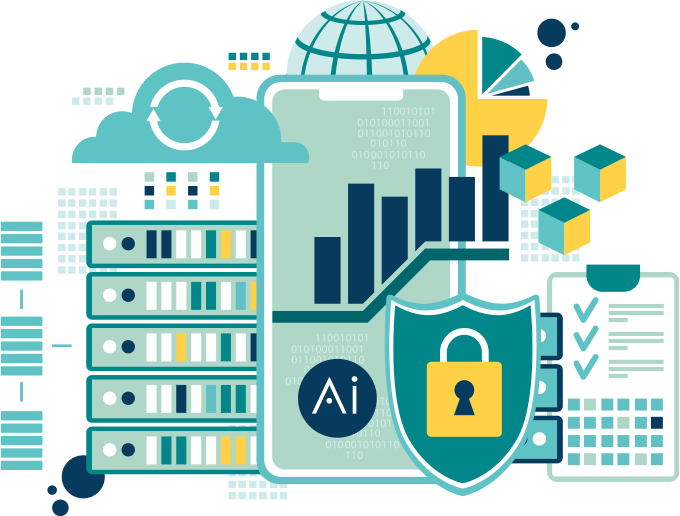
Optimizing work using technology.
For as many years as Sci-Fi has been a genre, there has been a fear that machines, computers, and robots are going to take over the world. A far less fictional, and in many cases, fully-realized fear, is that as technology advances, it will replace humans in the workforce. While technology and machines are replacing certain aspects of human work, humans still provide the literal ‘life-blood’ of the future of work.
The Industrial Revolution created a workplace in which humans and machines worked together with regularity. This relationship, while often fraught, mostly allowed the human workers to perform with greater efficiency and accuracy than was ever possible before. In most cases, humans interacted with the machines directly, providing the guidance and instruction machines needed to complete tasks.
In recent years, there has been a technological boom in machine learning, which uses algorithms and statistical models to develop computer systems that can learn and adapt without constant human instruction. But while machine learning does eliminate the need for humans in certain aspects of work, it’s not removing the need for humans altogether. Now as in the past, humans are using the ‘intelligence’ of machines to increase their own overall competency and performance.
Machine learning aims not to replace humans, but to find ways to optimize the relationship between machines and humans. The ultimate goal is always to improve and expand business intelligence – which is the technical infrastructure for how a business collects, stores, and analyzes data. Professor Carsten Østerlund explains a citizen science project where scientists use machine learning to clean and analyze data collected en masse by volunteer citizen scientists.
I think it’s up to us to help design relationships that are developmental, not only for the machine but also the humans.”
“I’ve been working on a project where scientists use machine learning to clean data,” says Østerlund. “These astrophysicists are detecting gravitational waves, and their detectors are so sensitive that they produce a lot of glitches and mistakes. We have designed a project where volunteers train the machine to find these glitches. This is an example of machine learning helping scientists to save time. But, we can also use machines to help train the volunteers to understand more about the detectors and the glitches around astrophysics.”
In his project, which recently received a grant from the National Science Foundation, Østerlund and his team will build relationships between volunteers and machines in which both will learn. The volunteers for this project don’t necessarily have a background in astrophysics. Initially, the machine will show images to the volunteers that the machine has already verified, and is familiar with. As the volunteers start understanding the images, the machine will then show images that it is less sure about; thereby increasing the difficulty of the tasks for the volunteers over time.
“The volunteers get harder and harder tests, and when they get really good, they start looking for new types of glitches that the scientists don’t know about. And at that point, their relationship with the machinery changes completely because then they start using machine learning as a search engine,” explains Østerlund. “They might find 10 glitches they think belong to a new class. They give that to the machine and say, ‘Find me more like this.’ That’s a new kind of dynamic relationship between the machine and humans. That’s one of the things that I’ve been interested in trying to figure out. How can we design these relationships where both learn?”
Østerlund is also working on a second project with School of Information Studies Assistant Professor Ingrid Erickson and business intelligence analysts within the healthcare system. In this project, business intelligence analysts (actual humans) use data to predict different needs within the hospital, such as how many beds they need on a given day, or how many nurses they should schedule. The analysts can also observe the hospital’s financial health and determine where costs are too high, and where the hospital can make strategic cuts. Once the analysts understand the trends, they can train machine learning to create an automated dashboard that provides hospitals with real-time data, allowing departments within the hospital to make dynamic decisions for optimizing cost and care.
The relationship between machine learning and human learning transcends many industries. In banking, for example, machine learning helps banks understand their customers by predicting when they may need a loan, or when they may want to open a new investment account. Similar types of machine learning are also being deployed in other branches of personal finance. There are now ‘Robo-advisors’, designed for personal portfolio management. These greatly lower conventional barriers-of-entry for amateur investors to manage their own investment portfolios, and in these cases, the investments are aided by algorithms which run off input from the investor’s own goals and risk tolerance.
There are many ways in which humans and machines work together, but it’s also important to note that humans possess many skills that machines simply cannot replicate. “The future of work is not about platforms,” says Erickson. “Platforms are important to the future of work, but the future of work is not about platforms. One of the things you see on the rise about culture, particularly in the United States, is the need for care work. Not carers, but the role of care in the future of work. It will rise to be a special skill in the sense that machines can’t do that very well and probably won’t be able to do that very well.”
One industry that is relatively safe from workforce displacement is counseling and therapy. The elements of care that are required in this field can only come from another human, and machine learning poses no threat to those workers. However, machine learning is already being used to help clients when they are not in direct contact with their human support network. There are apps designed to keep and set medicine and appointment schedules, other applications are trigger detectors engineered to predict manic behaviors, and while these help humans in real time, they also bolster and improve business intelligence for the entire industry.
A persistent shortcoming of machines is that they struggle to analyze past data and put it into context for an evolving future. For instance, there are many companies that use hiring software to help them filter through applications, yet oftentimes the machine is trained to find applications with qualities similar to people who already work at the company. The problem is that the machine isn’t accounting for diversity, or unique skill sets. Instead, it’s returning applications that most closely match the current makeup of the company. This is why having a human working alongside the machine to check machine biases and anomalies in the data, remains an integral part of this process.
“We have to train people to know what to do when they face anomalies in data,” says Erickson. “We’re in a period of transition trying to understand how the parts play together and which things you can reverse engineer or problem-solve. That sort of skill set where you’re not relying only on automation, but what do we need to ask questions about?”
Ultimately, in terms of machine learning and human learning, the future of work isn’t so much about how machines will replace humans, but how machines and humans will work together to build a relationship that benefits the workforce.
“I think it’s up to us to help design relationships that are developmental, not only for the machine but also the humans,” says Østerlund. “It’s going to be a lot of work for us in building those relationships, but if we can, then we could get a much better machine and human relationship.”